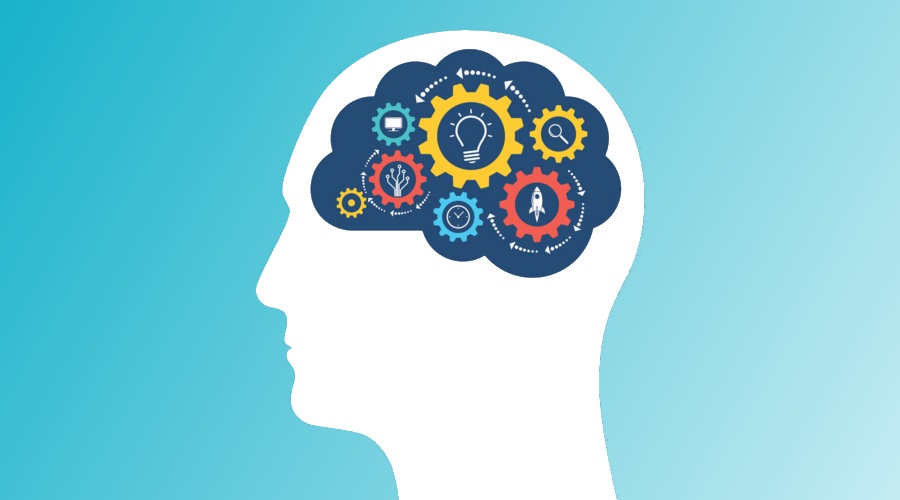
Providing great recommendations and product advice is no longer restricted to static knowledge models - instead, excentos combines guidance with artificial intelligence and learning algorithms. This creates the perfect mix of sales strategy into your Product Guide while adapting it to what your customers actually enjoy buying.
Many Guided Selling tools such as product advisors successfully "guide" the user through the product selection process. Firstly, they find out what the user requires and then recommend products that best fit to their specific needs. In most cases, product advisors exploit static knowledge models or use specific business rules in order to create and adapt to the recommendation behavior. Using this approach, the following advantages can arise:
- experts can define and assure the quality of the recommendation behavior, so you have everything under control (everything falls in line with the sales and marketing strategy)
- the recommendations can follow your sales strategy
- merchandising functions and push factors such as upselling, avoiding stockout situations, highlighting products with higher margins etc. can be implemented
Learning Helps Improving Product Guides
Algorithms that can learn the recommendation behavior from usage data have the following advantages compared to static knowledge models:
- ability to learn and adapt to buying trends e.g. changing trends in a fashion advisor or higher requirements for CPU power with evolving notebook technologies in a laptop advisor
- filling the gap where it is not possible or inappropriate to define the recommendation behavior instead of letting the users set the preferencese.g. for preferred colors, price ranges, brands etc
- less effort to (manually) define the knowledge model
- learning about dependencies where there is no product data
Combine Guidance with Preference Learning
Recent innovations of the excentos Lab allow combining the advantages of artificial intelligence-based learning algorithms with the guided approach of quality-assured knowledge models. Guided Selling is no longer restricted to static knowledge models - instead, we've developed recommenders that incorporate both approaches into one great sales and marketing tool:
- the basic recommendation behavior is still determined from a knowledge model-based approach. This ensures that certain quality criteria (e.g. a race bike must not be heavier than 10 kg) are met.
- depending on the use case, we let the recommender learn from usage data, i.e. every user's input, every transaction and also contextual information such as a user viewed a product, clicked on its image and so on makes the recommendations smarter.
There are numerous advantages of combining controlled guidance with flexible learning:
" self-learning Guided Selling tools are drastically increasing efficiency since they allow to control the recommendation behavior, but at the same time flexibly learn from usage data and optimize the conversion rate"
Examples where self-optimizing recommendations are required and boost shopping experience
There are a lot of industries where only the combination of the two approaches can guarantee great recommendations and a convincing shopping experience:
- fashion - e.g. make sure the right sizes are recommended to avoid unnecessary returns (knowledge model), and learn color/brand/style trends from usage data
- gift finders - e.g. make sure the right categories are displayed to the user, but learn which products have the best conversion for which use case and age of the gift receiver
- technical products - e.g. learn about the willingness to pay and typically accepted price ranges per target group, and learn which RAM size gamers like to buy for their laptop
- sports - e.g. make sure a running shoe fits the user's training goals (knowledge model), but learn what colors and brands users buy
- seasonal products - e.g. learn which products are bought around Easter or Christmas and make sure once Christmas is over, you don't stick to Christmas gifts only
Combine Learning With Guidance
Artificial Intelligence in Product Guides and recommender tools can significantly increase efficiency - however, they often lack appropriate expert knowledge and sales guidance. A combination of static expert knowledge models and self-optimizing recommendation behavior results in way more effective recommendations - so make sure your Product Guide supports both!